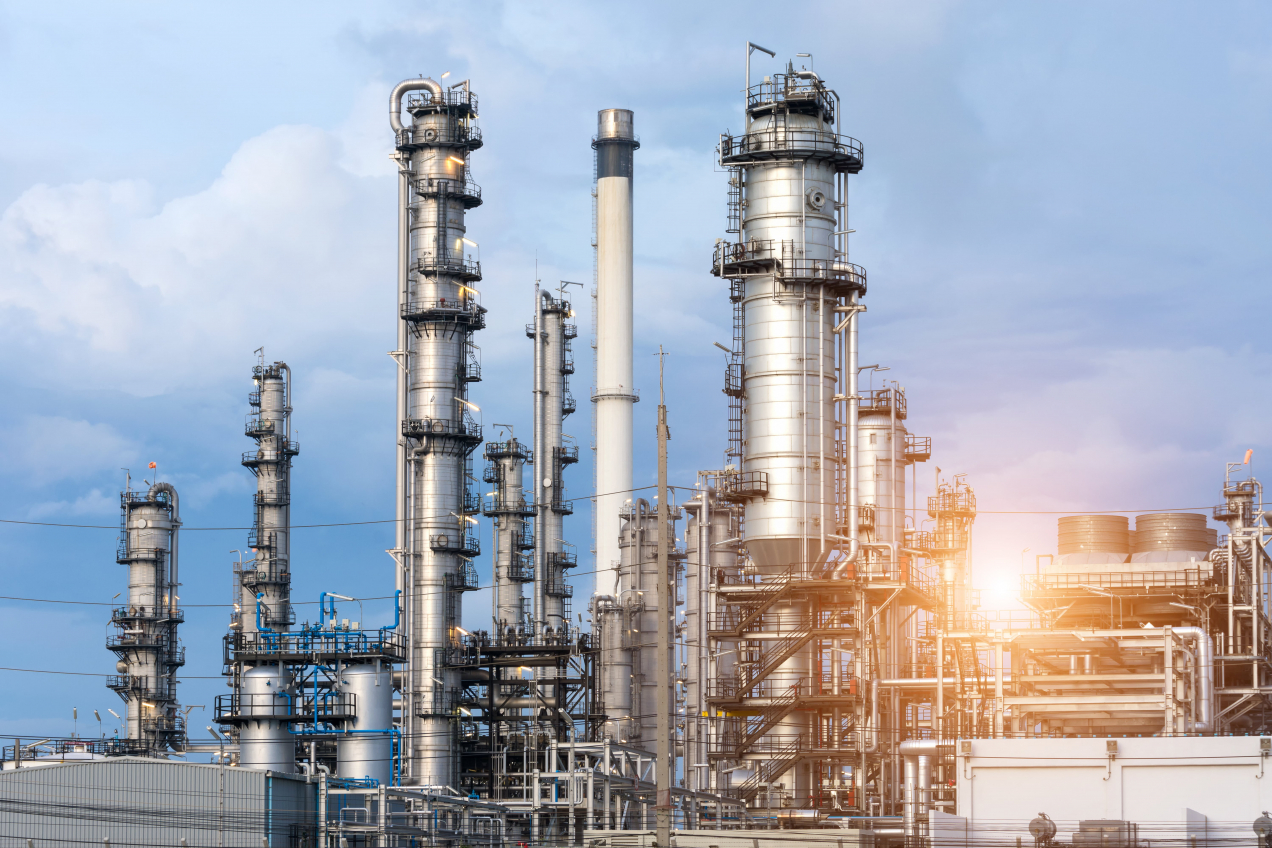
All industries have buzzwords. There are so many, in fact, that some years ago, there was a game designed to be played in boring overlong meetings called “Buzzword Bingo.” But sometimes buzzwords transcend the ordinary and become candidates for buzzword-of-the-year and even buzzword-of-the-decade.
That would be the concept of the “Digital Twin.”
For over a century and a half, when asset owners spoke of their plants, they meant the physical plant—the structures, piping, pumps, compressors, instruments, valves, conveyors, and all the rest that make up the infrastructure of the plant or manufacturing operation. Close to twenty years ago, people began to realize that there was an entire other structure in and about the plant. It was made up of all the data coming from the plant. Some of this data was process data, like flows, pressures, temperatures, and so forth; some was operational data like throughput, and some was data about how the plant itself was operating—like vibration, cavitation, valve stiction, and more.
Some very advanced asset owners realized that if it were digitalized, this structure was a very high-fidelity digital model of the plant in real time. They called it a “digital twin” in the wider framework of Industry 4.0.
OK, so what’s a digital twin?
According to Gartner, a digital twin is a digital representation of a real-world entity or system. The implementation of a digital twin is an encapsulated software object or model that mirrors a unique physical object, process, organization, person, or other abstraction.
Digital Twin technology produces an exact replica in software format, of a process, a product, or a service. Basically, it takes real-world data about a physical object or system as its inputs and produces outputs in the form of predictions or simulations of how that physical object or system will be affected by those inputs, and therefore how the physical object or system will act in response.
Data from multiple digital twins can be aggregated horizontally for a composite view across a number of real-world entities, such as a power plant or a city, and their related processes or vertically from the field level to the unit level in a single entity.
“The concept is exciting, absolutely, but more complex than one can be led to believe. Today there is a naiveté in many companies about the cost and time aspects,” says Marc Halpern, a Gartner Analyst.
So, it sounds great as a buzzword, and in articles and speeches about Industry 4,0, but when operators and engineers try to create and use a digital twin of their plant, the complexity often kills the project.
How do you use a Digital Twin?
A Digital Twin is made of amorphous clouds of data, so it is instructive to look at use cases.
First, Digital Twins can be organized into types or levels, and these can have different use case scenarios. Breaking the Digital Twin down into levels makes it much more understandable with much less handwaving and much more clarity. Starting from the bottom, with the least complex systems and moving toward more complexity makes the concept of a Digital Twin simpler and allows us to see the use cases more easily.
Let’s start with a very simple example. You can have a Component Twin. This collection of data is simple. You could have a twin for a piston, with bearing, and shaft. Being able to model such a simple set of hardware gives you the ability to collect data like oil temperature, piston speed, shaft vibration, bearing temperature, and with that data you can begin to predict when wear and failure may occur. This is how predictive maintenance begins.
Like a set of building blocks, we can increase the complexity of the Digital Twin by moving up to an Asset Twin.
This is a digital representation of an entire asset, for example a turbine, a motor, a compressor, or a control valve. Other assets can also be represented by a digital twin. In this model, you can see the aggregate of the information from the Component Twins that make up the Asset Twin. Asset Twins allow you to predict the failure modes the asset is subject to, and the failure lifecycle of the asset.
In addition to better operations and supporting predictive maintenance, asset twins can support product management, assist sales to gather knowledge on customer’s preferences and actual usage of their product and provide new service business models to drive revenue for the manufacturer of the asset. That same data, looked at from the asset owners’ perspective, can provide information to the asset owners who are trying to make sure they have the best possible assets and are operating them in the most efficient way.
Once you have your asset twins operating properly, and accurately predicting operation, maintenance requirements, and failure modes, you can start aggregating asset data in the larger system the assets operate inside. This next level would perhaps be a System or Unit Twin; for example, a Crude or Reverse Osmosis Unit. At this level the Twin helps product designers, architects, and engineers to improve future product versions and engineering models to optimize product performance and efficiency, accelerating time-to-market. At this level, a System Twin could be used to train operators and maintenance techs to recognize failure modes and determine what predictive maintenance techniques should be used to keep the unit operational, just as the Twin itself is being trained to improve its predictive ability.
Next level up would be a Process Twin. For example, a Composite Manufacturing Process. At this level, a Digital Twin helps management to get new operational data feeds into production and planning models thus paving way for strategic insights, recommendations, and road maps, not to mention coordinated predictive maintenance plans for the entire plant.
What Makes a Digital Twin?
Digital Twins consist of four essential elements:
- First is the physical equipment – the actual equipment item or items that you are interested in creating a twin for, to the lowest level you want to model—down to the component level. The higher the resolution of the physical equipment descriptions, the higher fidelity the model will be. Let’s say you want to create a digital twin of a motor. You need the information on the nameplate, how many hours the motor has run since the last maintenance, and a baseline vibration value. Anything else that can help you describe the motor to very high resolution is helpful in making the digital twin.
- Next is the Twin Model – The software model consisting of a hierarchy of systems, sub-assemblies, and components that describe the twin and its characteristics enriched by asset, operational, historical, and context data. The more operational and historical data you can gather from prior history, the easier it is to build the model.
- In addition, there is Knowledge – Data sources that feed the twin with operational settings, domain expertise, historical data, and industry best practices. These sources include sensors, calculations, data imported from other databases, and from the motor control center.
- Finally, you must have the Analytics – Simulation and/or Machine Learning models: these can be physics-based models, statistical models, and machine learning/AI models to help describe, predict, and prescribe the behavior (current and future) of the asset, system, or process. With products like UReason’s APM Studio, these models can be easily built by operations and engineering personnel.
How Do You Use a Digital Twin?
If you listen to the hype, Digital Twins will solve most maintenance and operations problems. This, like many other things, is just too good to be true. A Digital Twin is a powerful tool to visualize, simulate, and predict the operation of an asset or component, or even of a process train or manufacturing assembly plant, but it is only an Industry 4.0 tool. In order to use a Digital Twin, you also have to have expertise in operating your plant, expertise in maintenance and repair operations, and access to as much operating history data as you can get.
Sometimes, a Digital Twin just isn’t the right tool for the job. So here are some criteria to consider for Digital Twins so you can institute and enhance your data-driven maintenance goals.
- Can you visualize or otherwise specify the problem you need to solve? You need to have domain experts who clearly understand the problem, and who can use their expertise to decide if the problem can be solved by using a Digital Twin to simulate and predict the outcome.
- Can data from the field level be extracted and enhanced to provide further insights higher in the chain?
- Do you have sensors that not only give you Process Variables (PV) but also other data like changes in pressure in a line, or cavitation or excess vibration in a motor or pump? Do you have virtual sensors that can be created with data from the process you are working on?
- Does the field level data provide input to the existing operational history of the equipment? Does the data show both good and bad outcomes? Both types of outcomes are necessary for training the Digital Twin’s AI to make decisions.
- Finally, the problem you are trying to solve must be in support of a business case. There should be a target, or an outcome to predict or calculate that is of value to operations. Value could be reduction of planned maintenance and replace it with predictive maintenance, creating longer preventive maintenance time horizons, knowing hourly/daily what the risk and associated cost of operation is, or simply the ability to run the plant at over 100% of capacity.
Once you’ve understood your problem, the next step is to define, build and realize the Industry 4.0 Digital Twin. Starting from the component level, build your twin all the way to the highest level possible in your plant, perhaps to unit level or beyond. You need access to all the data streams that define your model, your Digital Twin, and you need all the history you can find.
The next step is to run the model and see how close the operation of the Digital Twin is to the operation of the real-time physical asset. Then you begin an iterative operation, very much akin to “tuning” a control loop. This operation enables you to train the Artificial Intelligence (AI) built into software like UReason’s APM Studio. The better the AI is trained, the more accurate the predictions from the Digital Twin will be.
UReason’s APM Studio can be used to create Digital Twins at any level in your plant and makes it easy to combine and concatenate these twins to give you a well-made Digital Twin of your entire plant. It isn’t Industry 4.0 Buzzword Bingo; it is a fully realized tool to quickly implrement predictive maintenance and something operators and engineers can really use.
Download E-book
Check Out More of Our Articles
If you enjoyed reading this article and you want to learn more about the OI4 Demonstrator make sure to check out more articles on our website!